Cognitive Underwriting: Reimagining insurance processes with AI/ML
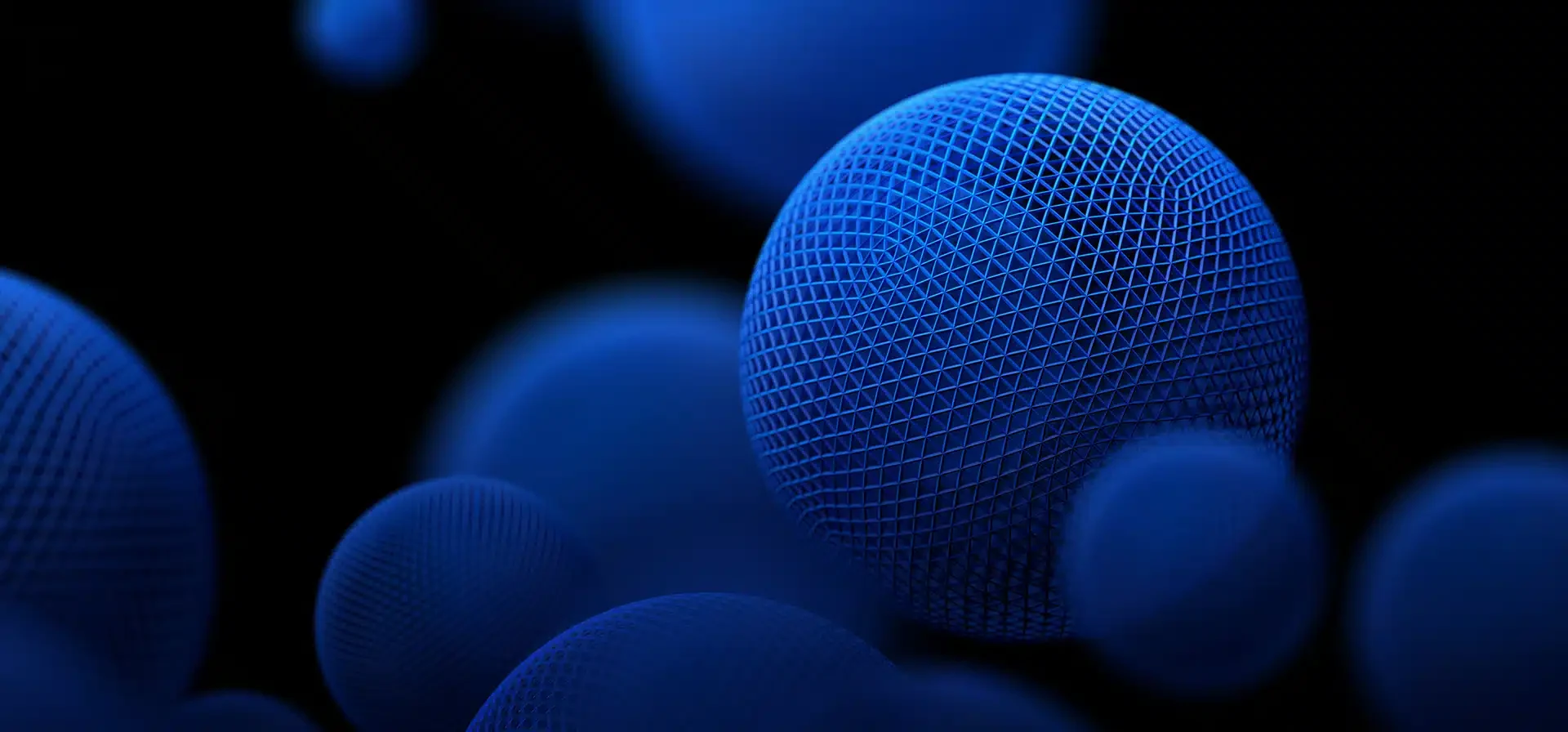
Underwriting decision-making has a significant impact on insurers’ business risk and profitability. The criticality of assessing and pricing risks effectively makes experienced underwriters a coveted (and expensive) resource. However, most underwriters today are struggling with mountains of data and administrative requirements. They waste precious time on incomplete applications, back and forth between multiple parties to collect information, non-premium-related endorsements, and often cumbersome manual analysis. According to studies, underwriters spend almost 70 per cent of their time on non-underwriting activities. The result?
- Longer application processing times
- Limited number of applications that an underwriter can process
- Higher chances of errors, inefficient pricing, suboptimal loss ratios
- Inefficient use of time for expert talent
- Delays that affect customer experience
- Risk of losing out the business to a competitor
It’s clear that the current state of underwriting leaves much to be desired. But can anything be done to change that?
Transforming underwriting with AI-led automation
McKinsey found that underwriters spend 30 – 40 per cent of their time on administrative tasks, such as rekeying data or manual analysis. Automating these tasks and deploying rule-based AI engines for key processes can drastically improve underwriting efficiency.
Case in point: New Business Intake
Underwriting new policies is a document-intensive process. Underwriting teams need to wade through volumes of varied and complex structured and unstructured documents to get insights that help pricing decisions. What if this whole process was automated? What if an AI-engine parsed these documents when new applications came in and extracted relevant insights? What if the AI engine could decide if an application is incomplete and automatically route it back for more information? What if conversational AI interventions could reduce the communication time between different stakeholders?
Today, all of this is possible. Automated data extraction, validation, and entry is helping insurers reduce the average handling time for new business intake by 5-10x. More efficient underwriting also means that the teams can process more applications and convert more new business.
Case in point: Endorsement
Similar benefits are apparent in endorsement processing for existing policies. Automating the indexing, classification, and data extraction of endorsement requests could save valuable time in re-issuing the policy. In addition, even non-premium-bearing endorsement requests such as address change, name change etc., are currently routed through underwriting teams. In fact, almost 50-60 per cent of endorsement requests for P&C insurers are non-premium bearing. An AI engine can easily identify and process these requests without involving the underwriters, as there is no need to recalculate premiums.
Improve business outcomes with cognitive underwriting
The impact of AI is not limited to operational efficiency. Beyond reducing the time taken for administrative tasks, AI can augment underwriting decision-making. For example, AI engines can pull data from third-party sources – that were previously inaccessible – to analyze an application’s risk better. They can even analyze the application data and match it with historical data to generate pricing recommendations with a higher chance of conversion. Insurers enabled with ascertainment of broking partner performance, can use feedback and right-partner fit to generate new business. Rid of the administrative burden and empowered by deep insights, underwriters can make better pricing and risk decisions that boost profitability and customer satisfaction.
Case in point: Broker partnerships
Inefficiencies from the broker’s end can result in delays, duplication of work, and loss of new opportunities. What if brokers could be given feedback on improving their document submission? What if brokers performing well could get leads on new and emerging opportunities? Timely insights from various broker interactions gives insurers the opportunity to monitor broker performance, provide real time feedback, and reward performance.
Case in point: Pricing
Right pricing is key to business profitability. Underwriters can use modeling based on analysis of historical internal and external data to arrive at pattern recognition. This allows for the grouping together of similar policies and similar risks, which can be leveraged to arrive at the right premium. For example, the use of parameters such as historical under-pricing resulting in high claims pay-out would categorize certain submissions as high-risk. When a similar submission is received, underwriters would receive real-time inputs on the risk upon data capture and model plugging. This enables data-backed decision-making around pricing, risk absorption, and conversion.